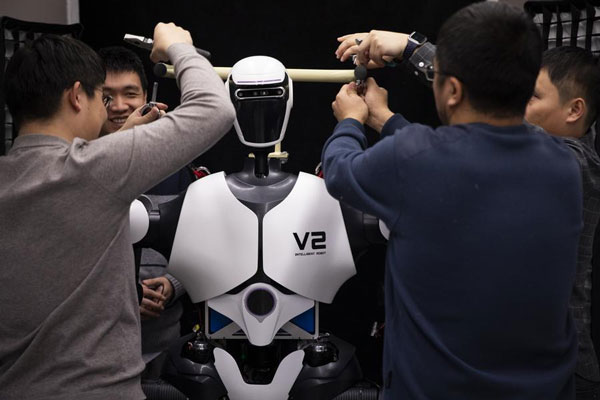
Feature: Artificial intelligence (AI) is a transformative force that is reshaping various aspects of our lives.
Let’s explore how AI is making an impact:
- Automation and Augmentation:
o AI can be categorized into two main areas: automation and augmentation.
o Automation involves using AI to replace human labor, streamlining processes, and reducing manual work.
o Augmentation refers to enhancing human capabilities by leveraging AI to improve decision-making, creativity, and performance.
- Applications Across Sectors:
o AI is already revolutionizing several sectors:
- Finance: AI algorithms analyze market data, optimize trading strategies, and detect fraud.
- Healthcare: AI aids in cancer screenings, drug discovery, and personalized treatment plans.
- National Security: AI assists in threat detection, intelligence analysis, and cybersecurity.
- Smart Cities: AI optimizes traffic flow, energy consumption, and urban planning.
- Transportation: Self-driving cars and efficient logistics benefit from AI.
- Criminal justice: AI helps predict crime patterns and improve law enforcement.
- Radical Advancements:
o AI is becoming more powerful and cost-effective.
o What was once computationally impossible or prohibitively expensive is now widespread.
o AIs can manage tasks like organizing events, developing business strategies, and designing cancer-fighting drugs.
- Challenges and Recommendations:
o Challenges include data access, algorithmic bias, ethics, and legal liability.
o To maximize AI benefits while safeguarding human values,
- Encourage data access for research without compromising privacy.
- Invest in unclassified AI research.
- Promote digital education and workforce development.
- Create advisory committees for AI policy recommendations.
- Engage with local officials to enact effective policies.
- Address bias complaints to prevent historical injustices.
In summary, AI is already transforming our world, and its potential is boundless. As we embrace this technology, we must balance progress with ethical considerations.
Ethical concerns related to AI
Ethical concerns related to artificial intelligence (AI) are crucial to address as this technology becomes more pervasive.
Here are some key areas of concern:
- Bias and Fairness:
o AI systems can inherit biases from their training data, leading to discriminatory outcomes.
o Addressing bias requires diverse data, transparent algorithms, and ongoing monitoring.
- Privacy and surveillance:
o AI-powered surveillance systems raise privacy concerns.
o Balancing security with individual rights is essential.
- Autonomous Decision-Making:
o When AI systems make decisions (e.g., in healthcare or criminal justice), accountability becomes complex.
o Ensuring transparency and human oversight is critical.
- Job Displacement:
o Automation may lead to job losses.
o Reskilling and policies to support affected workers are necessary.
- Existential Risks:
o Concerns about superintelligent AI endanger humanity.
o Research on safety measures and ethical guidelines is ongoing.
- Ethics in AI Development:
o Developers must consider societal impact, safety, and long-term consequences.
o Ethical guidelines and codes of conduct are essential.
- Autonomous Weapons:
o AI-powered military weapons raise ethical questions.
o International agreements are needed to prevent misuse.
- Transparency and Explainability:
o Users should understand how AI systems arrive at decisions.
o Explainable AI methods are crucial for trust.
- Data privacy and consent:
o AI relies on vast amounts of personal data.
o Clear consent mechanisms and data protection laws are vital.
- Social Manipulation and Deepfakes:
o AI-generated content can deceive and manipulate.
o Awareness and countermeasures are necessary.
Remember that addressing these concerns requires collaboration among policymakers, researchers, and industry stakeholders.
How can we mitigate bias in AI?
Mitigating bias in AI involves several strategies:
- Diverse and Representative Data:
o Collect diverse data from various sources and demographics.
o Ensure representation across gender, race, age, and socioeconomic backgrounds.
- Preprocessing and Cleaning:
o Remove biased labels or features from the training data.
o Regularize models to reduce overfitting to biased examples.
- Fairness Metrics:
o Define fairness metrics (e.g., demographic parity, equalized odds).
o Evaluate models using these metrics during development.
- Algorithmic Techniques:
o Use techniques like reweighting, adversarial training, and fairness-aware loss functions.
o Adjust the model predictions to achieve fairness.
- Explainable AI (XAI):
o Understand how models make decisions.
o Explainable models help identify bias sources.
- Human Oversight and Auditing:
o Involve domain experts to review model outputs.
o Conduct regular audits to detect bias.
- Regular Updates and Monitoring:
o Continuously monitor model performance.
o Update models as new data becomes available.
Remember, bias mitigation is an ongoing process, and collaboration among researchers, policymakers, and practitioners is essential.
Examples of bias in AI systems
Biases in AI systems can arise from various sources, leading to discriminatory outcomes.
Here are some real-world examples:
- Amazon’s Gender Bias:
o Amazon has developed an AI-driven hiring tool to screen job applicants.
o However, the system exhibited gender bias, favoring male candidates over female ones.
o The bias stemmed from historical data, which predominantly featured male applicants.
- Facial Recognition Bias:
o Facial recognition algorithms have shown racial bias.
o For instance, some systems perform poorly on people of color due to underrepresentation in training data.
o This bias can lead to misidentifications and reinforce existing inequalities.
- Criminal Justice Algorithms:
o AI tools used in criminal justice predict recidivism and sentencing.
o Some systems exhibit racial bias, disproportionately affecting people of color.
o Biased data and algorithmic decisions can perpetuate unfair outcomes.
- Loan Approval Bias:
o AI models for loan approvals may unintentionally discriminate.
o Historical lending data can introduce biases against certain demographics.
o This affects access to credit and economic opportunities.
Remember that addressing bias requires ongoing vigilance, transparency, and collaboration across disciplines.
Do you have an advertisement or article you want to publish? Mail us at theugreports@gmail.com or WhatsApp +256757022363.